Associate Professor Marco Caricato named February 2022 Sutton Family Research Impact Award recipient
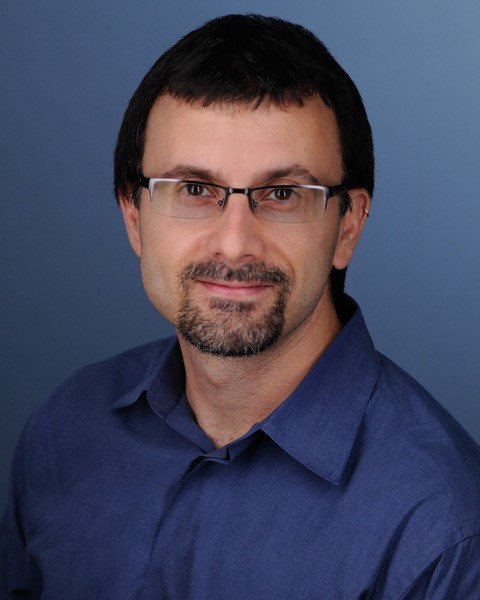
The Department of Chemistry congratulates Associate Professor Marco Caricato on receiving the February 2022 Sutton Family Research Impact Award!
The Sutton Award is a monthly competition among chemistry faculty. Every month, the Chemistry Department Chair and Associate Chairs review the peer-reviewed papers published by chemistry faculty from the three previous months to select a winner. The recipient receives a $500 cash prize and is featured on the departmental website.
For a full list of winners, visit our Sutton Family Research Impact Award webpage.
About the winning research
Winning paper: Cluster Model Simulations of Metal-Doped Amorphous Silicates for Heterogeneous Catalysis
This work discusses how cluster models can be effectively used for quantum chemistry simulations of metal-doped amorphous silicates. These materials have been successfully used as heterogeneous catalysts for a variety of reactions, including olefin metathesis and polymerization, and alcohol dehydration. The amorphous surface provides a large surface area and distorted metal sites that are very reactive. However, the disordered microscopic nature of these silicates makes them hard to characterize, and progress toward better catalysts relies on a trial-and-error approach. Using cluster models, it is possible to create multiple replicas of the same site with different distorted structures and silica environments, such that one can obtain a more realistic picture of the behavior of the material. These models allow to identify important structural descriptors of the active sites as well as a number of potential issues in the interpretation of experimental data. This work also discusses what challenges are still open in the simulation of amorphous materials and how these may be addressed in combination with new approaches such as machine learning algorithms.