Associate Professor Helena Malinakova named February 2025 Sutton Family Research Impact Award recipient
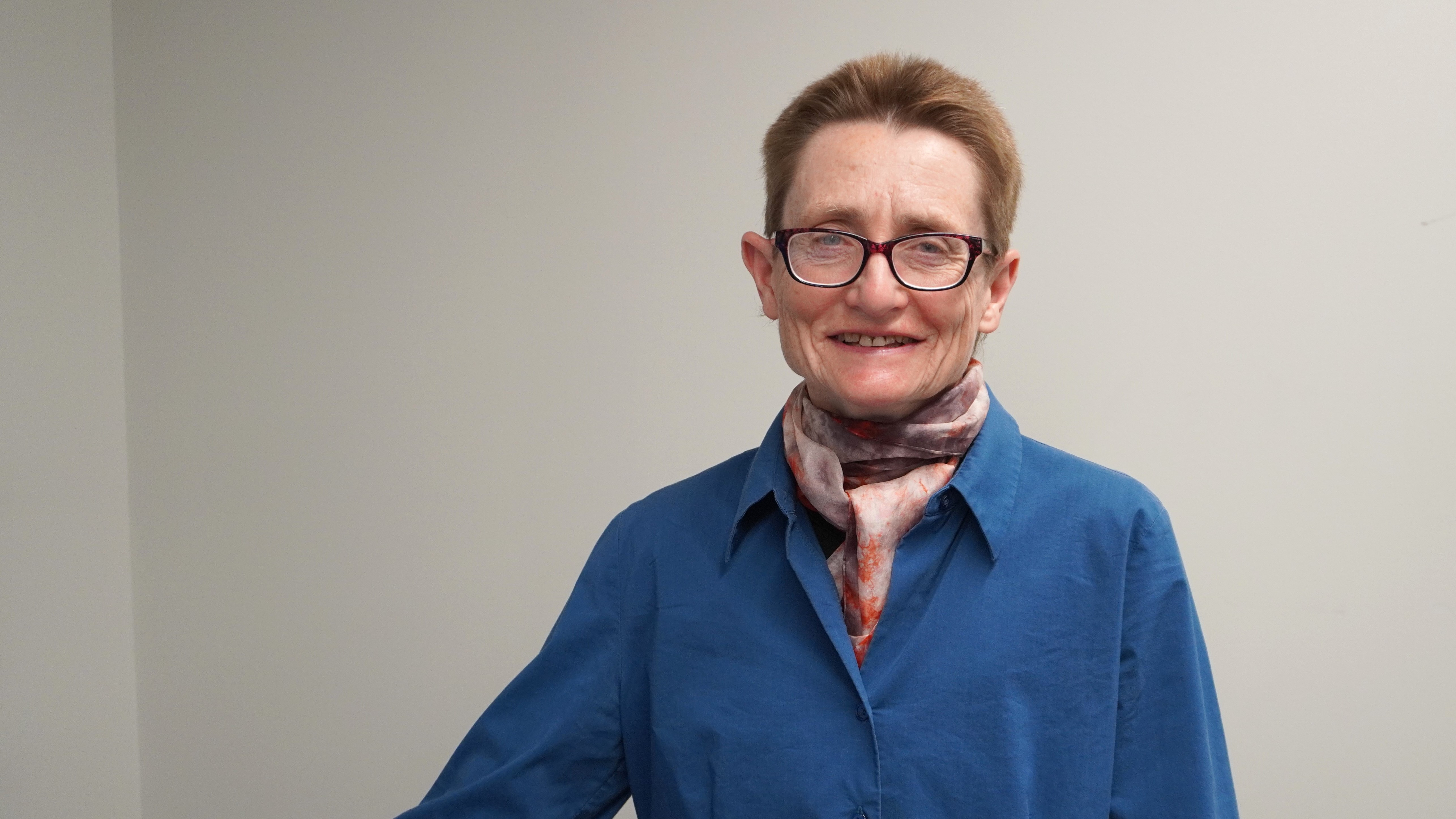
The Department of Chemistry congratulates Associate Professor Helena Malinakova on receiving the February 2025 Sutton Family Research Impact Award!
The Sutton Award is a monthly competition among chemistry faculty. Every month, the Chemistry Department Chair and Associate Chairs review the peer-reviewed papers published by chemistry faculty from the three previous months to select a winner. The recipient receives a $500 cash prize and is featured on the departmental website.
For a full list of winners, visit our Sutton Family Research Impact Award webpage.
Longitudinal Study of Students’ Study Approaches in Organic Chemistry Classes: Using Structural Equation Modeling (SEM) to Detect a Response Shift in Data from the OCH-Adjusted M-ASSIST Instrument
By Helena C. Malinakova
J. Chem. Educ. 2025, 102, 1, 15-26. https://doi.org/10.1021/acs.jchemed.4c00631
Students often consider organic chemistry to be a challenging subject. The material presented in organic chemistry appears to students as quite unique and distinct from the more quantitative content in physics or even the general chemistry courses. Teaching experience tends to suggest that students struggle to identify and commit to effective study approaches appropriate for organic chemistry courses. About four years ago, I embarked on a research project with the goal to precisely define, quantitatively characterize and explore the evolution of study approaches that students of organic chemistry choose to employ.
In the initial phase, I have adapted for the needs of organic chemistry a research instrument used to assess students’ study approaches, creating a unique protocol called OCH-adjusted M-ASSIST. The protocol employs a series of questions that students answer on a 1-5 Likert scale (agree to disagree). The questions serve as indicator variables for a two-factor (labeled “Deep” vs “Surface“ Learning) structural equation model shown in Figure 1.
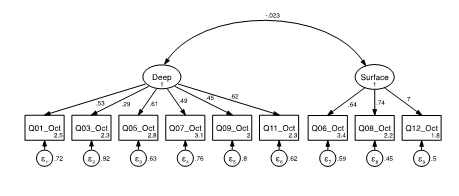
Figure 1. Structural equation model for students’ learning approaches in organic chemistry
The protocol assigns each respondent two numerical scores (1-5), the Deep and Surface learning scores, that indicate to what extent the respondent is utilizing the Deep (conceptual) and the Surface (memorization-based) learning approaches when studying organic chemistry. Our study demonstrated that students who achieved high grades in the course (A, B) increased their use of the Deep learning, whereas students who finished the course with grades C or lower increased their reliance on the Surface learning approaches when comparing their responses from the beginning (September) to the end (December) of the semester (Malinakova, H.C. J. Chem. Educ. 2022, 99, 2787).
In the recently published article selected for the February 2025 Sutton Award (Malinakova, H.C. J. Chem. Educ. 2025, 102, 15) I have explored in more detail how and why students adjust their study approaches in response to the very specific challenges encountered at about the half-point of the Organic Chemistry I course.
The data collected by the OCH-adjusted M-ASSIST instrument for the entire class (Organic Chemistry I) in October and December were found to fit well into the anticipated Structural Equation Models (SEM). The average Deep and Surface learning scores for the entire cohort of respondents failed to reveal any statistically significant change between the data from October versus December sampling. However, statistical comparison of the two SEM models by the chi-square difference (χ2DIF) test yielded notable differences between two key model parameters. A significant negative correlation between the Deep and Surface learning factors was only observed in the SEM model for the December data (see green highlighting in Figure 2). Furthermore, a statistically significant difference (called reprioritization) was noted exclusively in only one factor loading associated with the Deep learning factor (see the yellow highlighting in Figure 2). The specific item that was affected by the reprioritization was a question asking about students’ ability to “relate ideas” when studying organic chemistry. These two unique observations point to the true essence of the “organic chemistry challenge”.
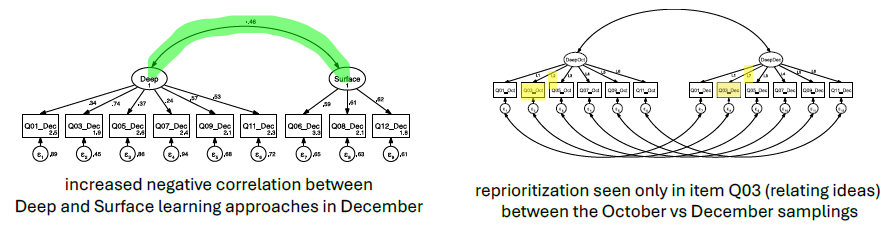
Figure 2. Statistically significant differences in parameters of the SEM models of learning approaches in organic chemistry (longitudinal study: October vs December sampling)
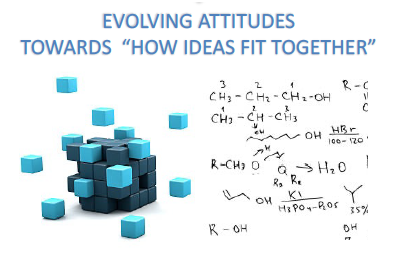
The data reported in this article demonstrate that the process of “relating ideas” is at the core of the challenge that students encounter in the first semester of the Organic Chemistry I course. Furthermore, it is in response to this challenge that students tend to refine their study approaches, gravitating either more strongly toward the Deep learning or toward the Surface learning approaches. These insights have both fundamental significance for the understanding of the learning process and practical implications for the designs of teaching methodologies. Methods and tools that would guide students more effectively through the “relating of ideas” process, and towards favoring the Deep learning over the Surface learning are most likely to elevate students’ performance in this challenging course.